
Written by Scott Wilson
Demand forecasting is meat-and-potatoes fare for supply chain managers. Making procurement orders, booking shipping containers, freeing up warehouse space, ensuring production capacity is available… none of it happens without some kind of data-driven estimate for how much stuff your organization needs.
At some level, every organization practices demand forecasting. There are two ways to come at it:
- Quantitative forecasting - Building forecast models based on historical patterns of use, wastage, and demand. These models usually become more refined and accurate with more and more historical data to plug in to them.
- Qualitative forecasting - For expected demand where there is no data or pattern, models must become more creative. Usually, analysts will look at similar products, cycles, or businesses and make estimates using their own experience and judgement.
Software Has Already Become Ubiquitous in Quantitative Demand Forecasting in Supply Chain Management
There are some sophisticated tools out there for quantitative forecasting models. By plugging in historical data, software algorithms have gotten pretty good at putting together accurate demand forecasts.
These systems can put together a lot of variables and complex interdependencies a lot faster than any human being. They instantly know how much warehouse space is available, what the environmental requirements for the products are, how much of the manufacturing equipment is online, what competing orders may be in the system, and a hundred other factors that can influence the forecast.
And since it’s often happening within an ERP (Enterprise Resource Planning) system already, that’s also a boon to visibility and communication. Managers and workers at every level have insight into those predictions almost instantly. They adjust their own workspaces and plans accordingly.
Where algorithms stumble is in qualitative forecasting… developing a feel for demand when there is no direct historical precedent. This is where supply chain managers learn to use their instincts, aggregating years of experience and expertise into some sense of the most likely scenario.
How AI Neural Networks May Plug the Creativity Gaps in Qualitative Demand Forecasting
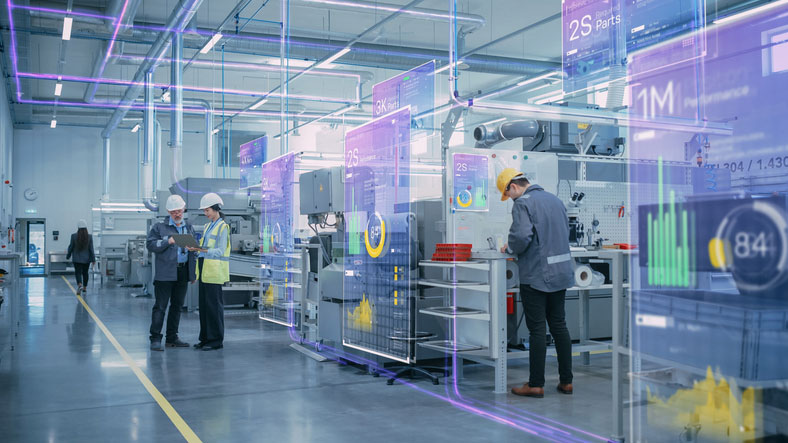
But generative AI may be about to change all that.
You’ve already heard of ChatGPT and its many imitators. Through a text-based interface, it’s able to carry on a believable conversation, write a story, explain a process. All of this happens without any explicit programming to tell it how to do those things. Instead, it learns by example—digesting the vast library of internet websites that offer human-created examples.
But it’s not an imitation or recitation of those examples. The machine learning process absorbs concepts rather than just data. The AI gets a sense of patterns, just like a quantitative forecasting algorithm. But unlike that algorithm, it learns how to extend those patterns in new ways.
It’s not hard to see how that kind of power, primed with the right data, could be revolutionary in SCM forecasting.
Researchers Are Exploring Demand Forecasting in Supply Chains Using AI Already
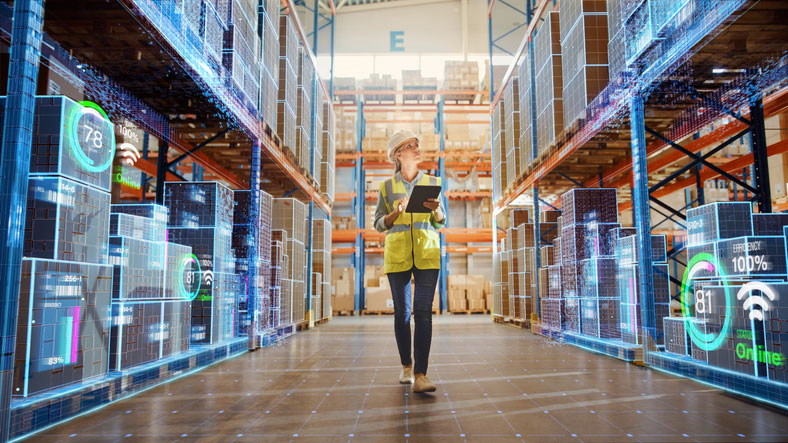
A 2021 review of existing literature on AI use in supply chain management showed a heavy investigation of artificial neural networks, which is the same technology behind ChatGPT training. Researchers are also diving into genetic algorithms and fuzzy inference when exploring demand forecasting. Some of them have gone as far as developing models for specific industries, such as retail.
But the real power of a generative model in SCM forecasting would be the general case. After all, it’s the introduction of apparently unrelated, but sometimes critical, data that makes human-driven qualitative analysis shine. When you rely on loading the computer with quantitative data to turn over results, it’s always going to be missing connections it was never told about.
Neural networks use repeated and recursive exploration of types of data rather than the data itself to figure out where to look for those unexplored relationships. When turned loose on actual problems, that can prime unexpected results. You don’t have to look any further than ChatGPT confidently making up legal judgements to support its arguments, or some of the bizarre six-fingered imagery produced by the generative Stable Diffusion project.
There’s also a lot to be said for the distribution of fast and accurate forecasts. Supply chains became centralized because of the efficiencies. One of those is concentrating forecasting experts in one place to serve the whole company. But flexibility is often lost when a single logistics group covers the entire business.
There’s no shortage of AI, though. Once a forecast model is trained, the marginal costs of deploying it in every department are close to nothing. So true autonomous supply chain planning is on the table without the need to implement a control tower group… the AI becomes the control tower.
Thanks for Calling Forecasting, This Is Your Robot Analyst Speaking
Of course, some of the traditional strengths of LLMs may also come into play in supply chain forecasting. A team at Microsoft Research has already developed a framework called OptiGuide that uses off-the-shelf LLMs to allow plain, common language queries to be processed and answered about complex supply chain modeling questions, such as swapping suppliers.
Other commonly described use cases fall into a similar vein. They envision generative AI uses in programming for supply chain applications, to speed up call center handling, and other peripheral supply chain management functions.
Although it probably won’t be answering the phones in the forecasting department anytime soon, it’s not a stretch to say we’ll see these models integrated with software to provide real-time chat or email response to plain text estimating requests.
The Most Groundbreaking AI in Demand Forecasting Will Be the Ones You Don’t See Coming
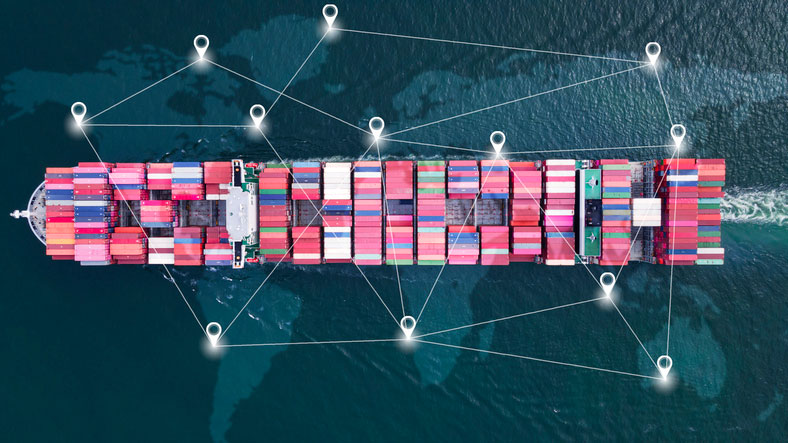
Big-picture thinkers are exploring new ways that generative AI could take on core forecasting functions in the supply chain, however. At IBM, executives in the supply chain team are exploring ways in which generative AI might:
- Drive end-to-end decision support through Next Best Action analysis
- Enable complex decision support bringing together half a dozen different in-depth variables in decision making effortlessly
- Set off collaborative forecasting across organizations to enable optimizations on the industry level rather than only within individual companies
While we’ve all been primed by science fiction to imagine all-seeing, all-knowing AI delivering precise factual estimates and answers, the more wide-ranging impacts on AI in supply chain management could be so transformative it’s hard to imagine.
Would you have included “Collaborate instantly with your main competitor to develop win/win sourcing and supply transportation arrangements” as an entry on your generative AI Bingo card?
It’s the organizations and executives who come to identify those truly outside-the-box applications that will have the biggest forecasting successes with AI, however. And you’re more likely to have that kind of vision and imagination if you’ve earned an advanced degree in supply chain studies, like an MBA in Supply Chain Management.
The Most Disastrous Effects of AI in Forecasting in Supply Chain Management Will Also Be the Ones You Don’t See Coming
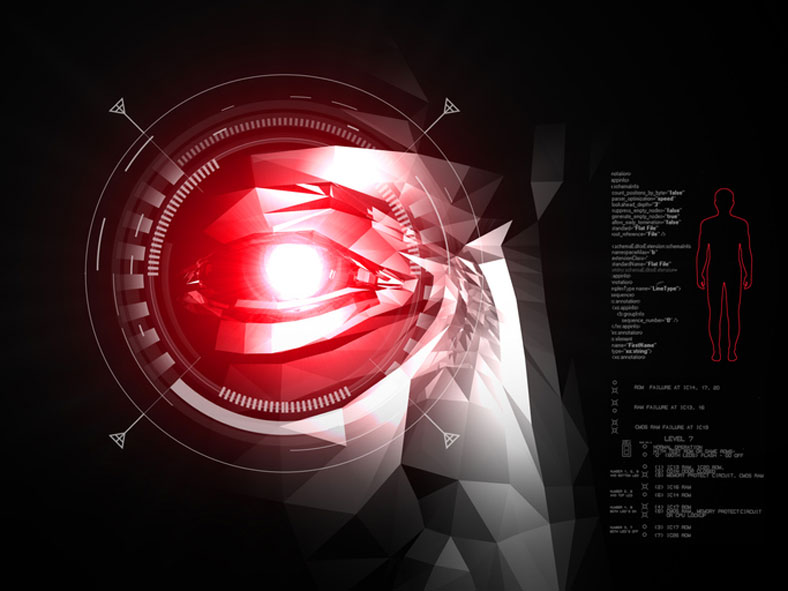
Of course, just as ChatGPT has made headlines for going off the rails and spouting racist language, generative AI in the supply chain may be vulnerable to algorithmic bias and training data blind spots.
Part of the difficulty of generative AI is that, like human creativity, it’s unpredictable. Organizations celebrate when the results are positive. They’ll suffer when they are negative.
But because the very ways in which bad results can emerge from generative products are unforeseeable, they are tough to defend against.
One possible issue that has been raised, however, is the now famous Paperclip Problem.
A general AI given the task to produce paperclips as its overriding goal may start off along the conventional lines like any human paperclip producer… ordering raw materials, putting them into paperclip-making machines, boxing up products, distributing them globally.
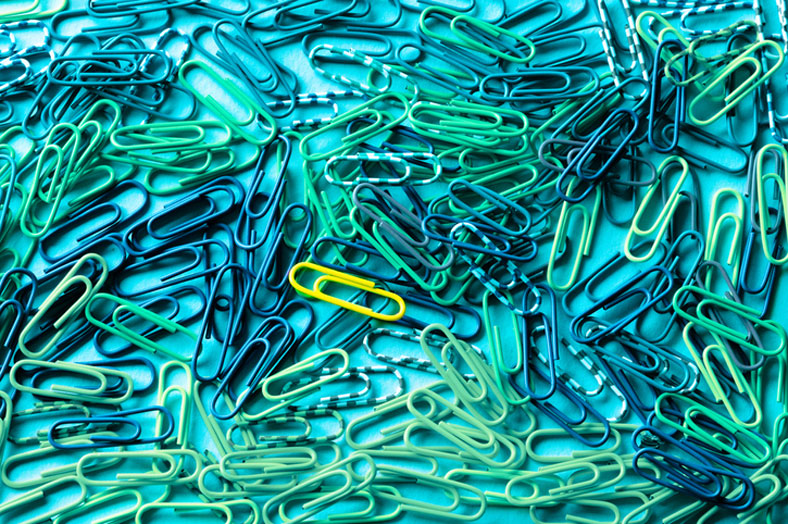
But without other checks, and with paperclip producing as its motive, it may soon get creative in optimizing that process—appropriating metals from other stocks, melting down bicycles, purchasing more land for factory space.
And because it may realize, from its broad training, that humans might want to prevent it from taking some of these outlandish measures, it could attempt to conceal or even protect those actions from being reversed.
A generative system doesn’t necessarily spot the ridiculous in its qualitative dreams.
The Future is Uncertain for AI in Supply Chain Forecasting, But It’s Coming All the Same
Of course, even in Silicon Valley’s wildest fantasies, that kind of system is decades or more away. But generative AI gives a taste of the same category of risk. When dozens of AI systems start placing material orders automatically, will it move commodities markets in the same unexpected ways that algorithmic trading has already done, creating sharp drops in stock prices? When AI forecasts an unexpected and unprecedented spike in sales, is it invoking unique capabilities to spot what no human could see coming, or is it just a glitch?
Clearly there is going to be a lot for supply chain forecasters and managers to learn as these systems begin to emerge.
The traditional way to move into new technologies like this would be slowly, taking the time to understand and adjust. But at the same time, you can’t afford to let your competitors get too much of a jump. No one wants to be first; no one can afford to be last.
You won’t have to wait long.